In the contemporary insurance landscape, the intersection of artificial intelligence (AI)-based predictive analytics and claims management is revolutionizing the way insurers operate.
The insurance industry, particularly in the realm of medical professional liability (MPL), has long grappled with the dual challenges of optimizing indemnity payments and controlling allocated loss adjustment expenses (ALAE), which includes those expenses incurred by the insurance company in the investigation, adjustment, or defense of specific claims. These complexities not only influence the financial performance of insurers but also the quality of service delivered to insureds.
Leveraging AI-based predictive analytics has the potential to propel the industry toward more informed and shared decision-making and strategic resource allocation. In the forthcoming article, you’ll gain insight into how AI claims analytics are used to reduce claims handling time and, hence, both indemnity and ALAE. That will help you understand the transformative potential of data-driven insights.
Leveraging AI as an Agent of Certainty and Productivity
The integration of AI-based predictive analytics into claims management workflows represents a monumental shift toward enabling heightened productivity within MPL. First, AI-driven systems excel at compiling benchmarking data, enabling carriers to compare their claims handling practices against industry standards and best practices, and identifying areas of strength and opportunities for improvement. Second, AI-based analytics can identify patterns that might elude claims professionals, facilitating more accurate risk assessment. Third, once closed, the claim’s outcome can then be fed into a machine learning model to improve the reserve accuracy for the next opened claim.
In addition, AI tools offer unparalleled claims management efficiency. They automate routine tasks, streamline claim processing, and minimize the likelihood of human error. The automation of portions of the workflow not only speeds up the claims handling process but also frees up human resources for more complex, value-added tasks without being a cause for layoffs in the industry. Additionally, AI-based tools, using natural language processing and large language model-related techniques, have the capability to summarize and interpret large volumes of reports and data. This attribute is invaluable throughout the claims process. They can quickly process and distill key information from lengthy claims documents, medical expert witness reports, and legal briefs, providing adjusters with concise, actionable insights.
AI-based claims predictive analytics have the potential to significantly enhance the communication channels between stakeholders such as adjusters, managers, insureds, and attorneys. For adjusters and managers, AI-driven analytics provide a common ground for decisions, ensuring no information falls between the cracks. When interacting with insureds, adjusters armed with AI analytics can offer clearer, more precise information regarding claim status and potential resolutions. Both parties are now better equipped for facts-based shared decision-making to consent to claims resolution. This transparency improves the trust and satisfaction of insureds as well as streamlines the communication process, reducing misunderstandings and delays. Lastly, communication is also facilitated with defense lawyers and experts as all can exercise appropriate and informed decision-making power.
How MPL Carriers Can Leverage AI Insights
The use of AI-based predictive analytics in insurance medical malpractice claims management paves the way for more granular investigations going forward. This is where additional value lies for individual carriers in benchmarking their track record against larger, customized MPL data sets. Analysis should also be done, on top of indemnity and ALAE, on other key metrics such as claim length and the percentage of non-zero loss claims.
By doing so, carriers can pinpoint their relative positions in the industry and identify areas ripe for optimization. Delving deeper with customized models tailored for individual carriers can increase productivity and enhance accuracy, especially when accounting for unique variables in different venues. This granular approach not only augments operational efficiency but also primes carriers for proactive strategies in an ever-evolving marketplace. The potential for innovation is vast for both insurance carriers and captives, inviting collaborations to continuously refine the nexus of AI-based predictive analytics and medical malpractice insurance claims management.
Optimizing Indemnity with AI-based Predictive Analytics
MPL claims tend to be complex and follow different patterns. In a generally stable environment, certain clusters of claims are still subject to high and/or rising indemnity payments. Outlying, sometimes hard-to-detect claims can still lead to devastating verdicts. Understanding the factors that drive indemnity will help carriers anticipate and enhance communication among stakeholders as well as close claims faster through the help of AI-based predictive analytics.
Observing historical data gives strong insights into certain trends driving indemnity. It is, however, only through the use of more advanced AI-based predictive analytics techniques that one is able to grasp the interrelational dynamics at play. These techniques allow carriers to elevate their analysis in various ways.
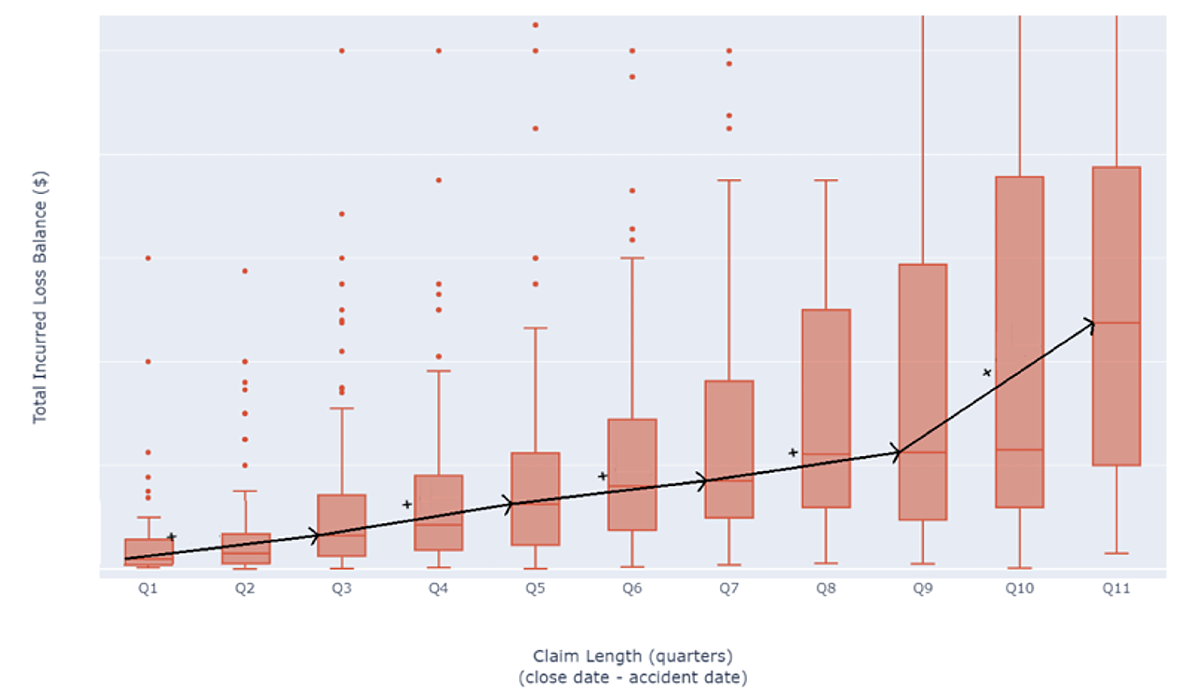
Observing the Trends: Challenging the inconsistency of indemnity starts with understanding the driving forces behind the amounts paid by insurance carriers. Whereas procedural elements carry a strong weight in the outcome of a claim, indemnity is also strongly correlated with the severity of the injury: Unsurprisingly, the more severe it is, the higher the indemnity.
However, one must be aware of exceptional behaviors: Solely emotional injuries tend to be more compensated than minor physical injuries, whereas death leads to lower compensation than very severe, yet not fatal, injuries, as seen in the figure below.
The profound influence exerted by injury severity warrants meticulous statistical analysis to discern the influencing factors that operate independently of severity. By adjusting for severity, certain noteworthy insights come to light. Specific facilities, particularly nursing homes, tend to experience lower indemnities. Similarly, certain medical specialties show very different indemnity amounts. For instance, as you might expect, obstetricians and gynecologists have much higher average indemnity amounts than other practices.
On the other extreme, dentists show the lowest indemnity amounts of all specialties. In this context, it appears that dentists display a higher tendency to offer indemnity settlements than their peers, leading to faster claim closures and more judicious costs. Understanding the stakes of a claim earlier on allows carriers and practitioners to make informed decisions about the handling of a claim, thus reducing exposure in the long run. However, as more and more factors kick in, it becomes difficult to accurately determine how a claim will evolve without the appropriate tools.
Sharing the Experience through Models: Several models were developed on various use cases, reaching optimal levels of accuracy in less than three months. Rich datasets, composed of structured and unstructured historical claims data from several MPL insurance carriers, allow for a deeper understanding of the influence of each factor inside a particular claim. Using predictive capabilities, models have been built to predict the outcome of both verdicts and settlements in order to help parties reach a fair agreement earlier on.
In the figure below, the lift curves show how close predictions are from the final outcome of claims in each range of indemnity award. Predictive models should consider historical data on injuries and procedures, using fine grain analysis on ICD-9 and ICD-10 codes, up to the most detailed levels of the classifications.
Models should also consider elements closely related to each healthcare practitioner, such as their specialty, practice type, board, and employment status, which constitute heavy drivers of indemnity models. All of these elements have been further analyzed for each jurisdiction as they carry predictive value, leading to outcomes with a 10x ratio on a priori similar claims. The powers of shared datasets and algorithms allow each and every single carrier to benchmark their track record with the rest of the market and deal in new markets as if they had decades of experience.
Optimizing ALAE with AI-based Predictive Analytics
As ALAE grows, carriers need tools for early prediction that can help in slowing down the increase, stopping it, or reversing it. Historical datasets reveal that ALAE has consistently tracked upward for both zero-loss and non-zero-loss claims. This persistent increase can be ascribed in part to factors beyond traditional measures of inflation, including evolving standards and burdens of proof and other statutory standards, as well as the intricate nature of medical malpractice claims.
Analysis of average ALAE by claim outcome reveals a noteworthy phenomenon: the average ALAE nearly doubles in cases where plaintiffs receive any indemnity payments—in other words, non-zero-loss cases.
Failure to effectively address burgeoning costs associated with escalating ALAE could pose a substantial threat to the stability of the MPL insurance market. This, in turn, may lead to diminished affordability and accessibility of coverage for healthcare providers. That underlines the imperative behind developing proactive risk management strategies capable of forecasting and mitigating the escalating trend in ALAE.
Unveiling the Drivers of ALAE: After regularizing any sample distribution, there is evidence that certain states consistently exhibit a notable correlation with higher ALAE than the average. The introduction of a variable that anonymized this variable unveiled crucial determinants of ALAE, shedding light on factors that exert substantial influence on its fluctuations. In this regard, three specific factors impact claim resolution strategy:1
- Type of allegation: The primary allegation, especially procedure errors, strongly correlates with ALAE, particularly in ob/gyn claims, influencing the complexity of the investigation process
- Injury severity: The severity is another critical factor, with higher severity levels generally leading to higher ALAE, although the highest severity level 9 (death) shows a lower ALAE than severity 8, which is known as grave or life threatening
- Provider specialty: The provider's specialty significantly affects ALAE, with neurological surgery and ob/gyn specialties showing higher ALAE, highlighting the variable complexities in claim processing across different medical fields.
Drive Toward Early Prediction and Mitigation in MPL
In the face of rising ALAE, AI-based predictive analytics emerges as a powerful tool for insurance companies and healthcare providers. By harnessing the capabilities of machine learning and predictive algorithms, organizations can enhance their ALAE forecasting, allocate resources more efficiently, and ultimately make informed business decisions.
The practical goal of AI-based predictive analytics in the realm of ALAE is twofold: early prediction and effective mitigation. By identifying high-risk specialties and primary allegations, medical malpractice carriers can proactively allocate resources, streamline investigations, and optimize negotiation strategies.
This not only helps in managing costs but also in ensuring timely and fair resolutions for claimants. Furthermore, AI-based predictive analytics allows insurers to anticipate the potential impact of close years, policy limits, practice types, and patient age on ALAE, paving the way for strategic decision-making.
By leveraging their data, carriers can anticipate receiving valuable returns at both the industry-wide and company-specific levels, including benchmarking their performance with industry best practices, more precise predictive models for assessing industry-wide trends, and tailored predictive models for managing claims, thereby preventing potential devastating verdicts. This comprehensive approach not only enhances the effectiveness of AI-based predictive analytics but also boosts the overall ROI of these endeavors, making them a strategic asset as well as a low-risk option for insurers.
Create Custom Predictive Models
Moving forward, optimal levels of accuracy can be achieved by incorporating additional information into predictive models. Whereas general data constitutes the base foundation of any stable and accurate model, claim handling sometimes requires more precise information. More granular information can be incorporated about each venue, judge, expert, and attorney, as well as contextual information contained inside adjusters’ notes.
Using automated natural language processing, machine learning, and generative AI, it is possible to structure data of different forms and create tailor-made prediction models. Those models will assist insurance claims professionals and their advisors, alerting them of potential devastating claims and consistently providing the fair value ranges to settle losses before unnecessary escalation. Implementation of such decision-support tools can lead to as much as 14% savings in incurred loss.
Evolve Strategies to Manage ALAE
As the medical professional liability landscape continues to evolve, so must the strategies employed to manage its financial implications. ALAE, as a significant component of these costs, requires thorough analysis and predictive insights to safeguard the stability of the MPL insurance market.
By understanding the intricate interplay between healthcare practitioner specialty, primary allegation, claim severity, jurisdictions, and other influential variables, insurance companies and healthcare practitioners can chart a course toward early prediction, efficient resource allocation, and shared decision-making. In an era defined by informed strategic vision, timely execution, and seamless communication, the marriage of AI-based predictive analytics and medical malpractice insurance holds the promise of a more stable and sustainable future for both insurers and insured.